Yingting Gao / 高莹婷
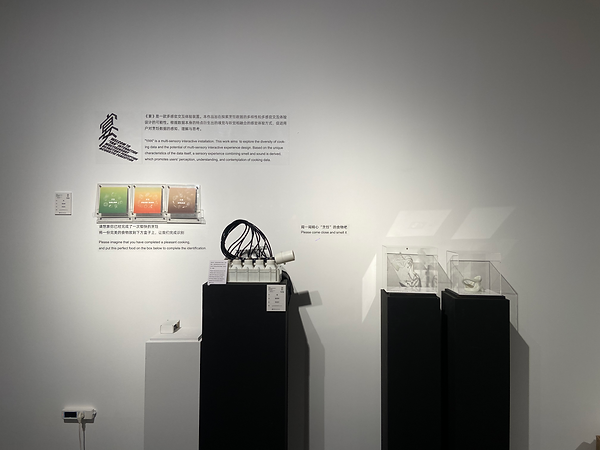
/ Introduction
​
Cooking, as a user behavior rich in sensory information, offers ample opportunities for exploring multimodal data and data interaction methods. Currently, the primary sources and interaction modes of cooking data still predominantly involve visual senses, while attention to olfactory, and audio senses remains limited. Therefore, this work explored the diversity of cooking data and the possibilities of multisensory interaction experience design by examining existing data collection methods, cooking experiences, and sensory sciences to propose an innovative multisensory interaction design and practice methodology that allows users to experience and interact with data from the perspective of digitized scents and sounds.
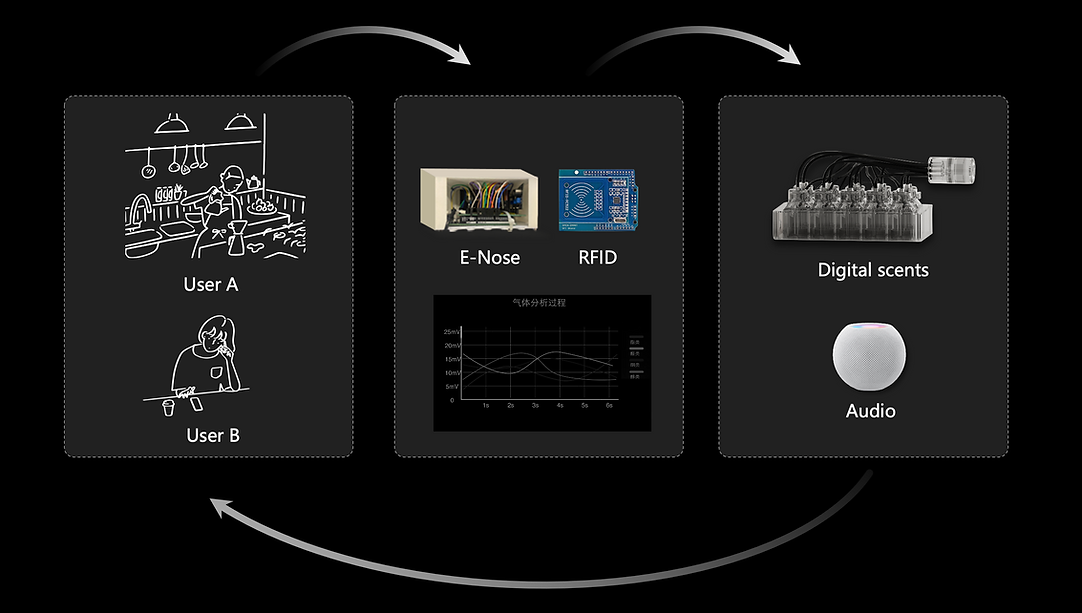
We designed an RFID system configured near the refrigerator to identify the type of ingredients taken by the user, and designed, and integrated, an array of five different types of gas sensors for detecting odor pattern and changes during the cooking process.
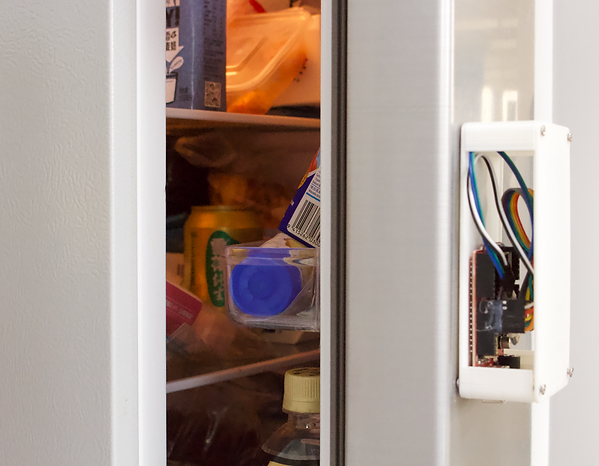
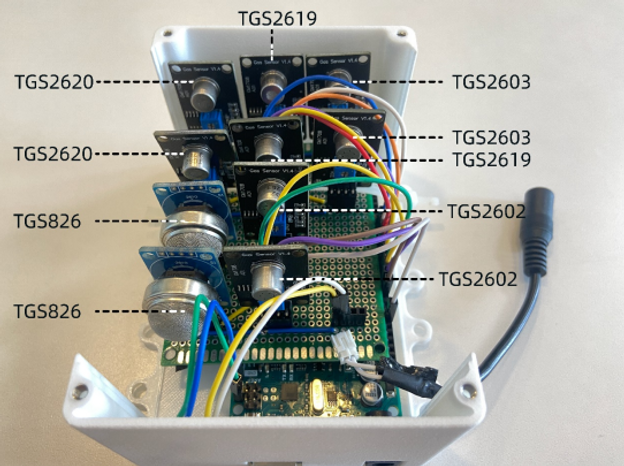
We used the gas sensor array to continuously monitor the gas generated during the cooking process of 83 dishes, and builds a classification model of cooking methods based on machine learning with an average accuracy of 95%. The following figures show the patterns of odor changes during cooking of three foods: boiled spinach, scrambled eggs and fried chicken, respectively.

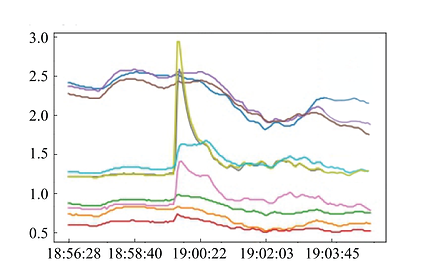
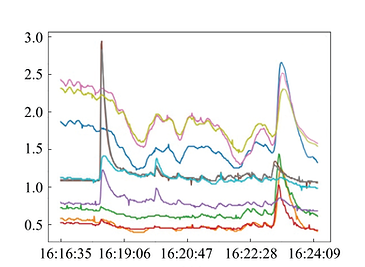

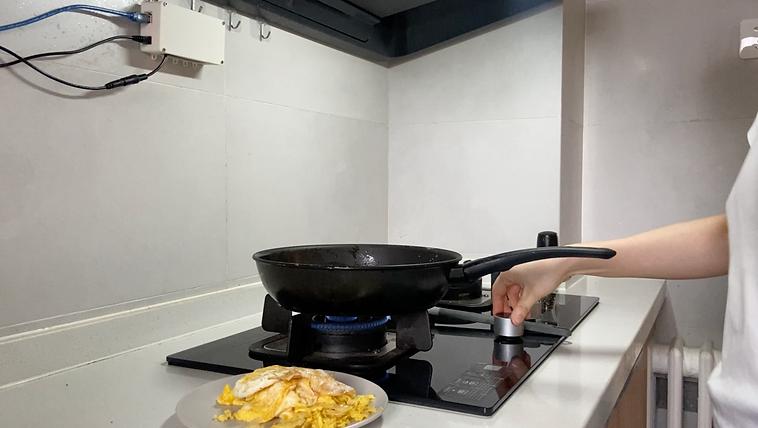
We used the gas sensor array to continuously monitor the gas generated during the cooking process of 83 dishes, and builds a classification model of cooking methods based on machine learning with an average accuracy of 95%. The following figures show the patterns of odor changes during cooking of three foods: boiled spinach, sautéed vegetables and fried chicken, respectively.
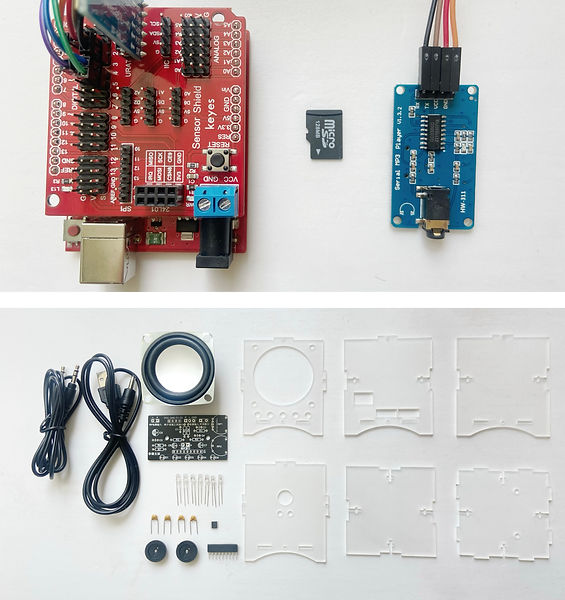
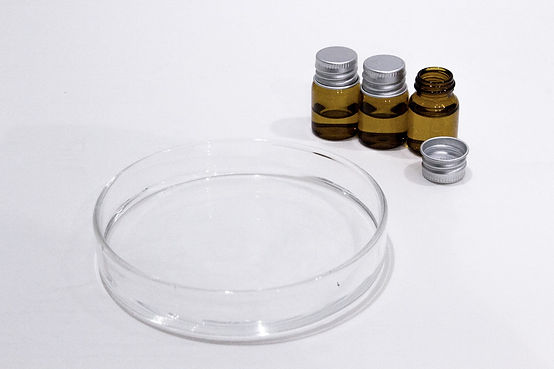
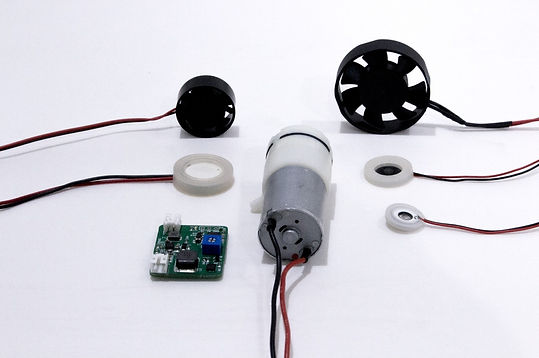
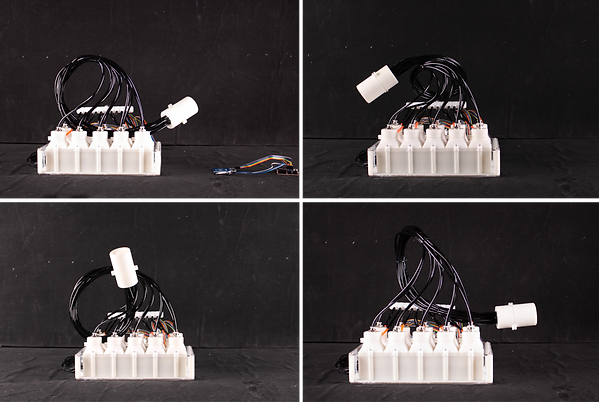
